In today's rapidly evolving technological landscape, artificial intelligence (AI) has become integral to various industries, including the banking sector. Banks and other financial institutions increasingly leverage AI systems like machine learning and data mining to streamline operations, enhance efficiency, and provide better customer services. However, the inherent complexity of AI models often leads to a lack of transparency, commonly referred to as the 'black box paradox'. This opacity raises concerns about fairness, bias, privacy, and ethical implications. To address these challenges and foster trust in AI systems, the concept of Explainable AI (XAI) has emerged.
Understanding the Black Box Paradox in AI
The black box paradox refers to the inherent opacity of AI systems, where the decision-making processes are often obscure and difficult to comprehend for humans. This lack of explainability makes it challenging to understand how AI arrives at its conclusions, leading to questions about the fairness and reliability of the system. Privacy and civil liberties groups have raised concerns about algorithmic bias, which can result in discriminatory outcomes, such as denying loans or services based on gender or ethnicity. These issues have prompted banks and financial institutions to explore solutions to address the black box paradox.
Introducing Explainable AI (XAI)
Explainable AI (XAI) is an emerging field that aims to make AI systems more transparent and understandable to humans. It provides the tools and techniques to explain the reasoning behind AI decisions, allowing auditors, analysts, and stakeholders to trace how these decisions are made.
By incorporating XAI, financial institutions can identify and mitigate biases, ensure compliance with regulations, build trust with customers and regulators, and unlock the full potential of AI technology.
The Benefits of Explainable AI in the Financial Sector
Implementing XAI in the banking industry offers several benefits, including increased productivity, trust building, surfacing new value-generating interventions, ensuring business value, and mitigating regulatory and other risks.
Increased Productivity
XAI techniques enable quicker error detection and identification of areas for improvement, enhancing the monitoring and maintenance of AI systems. By understanding the specific features that contribute to the model's output, technical teams can validate the applicability of patterns identified by the model and optimise its performance.
Trust Building
Explainability is crucial for building trust among customers, regulators, and the public. When the reasoning behind AI recommendations or decisions is transparent, customers can have confidence in the fairness and accuracy of the system. Sales teams, for example, are more likely to trust AI applications when they understand the basis for the recommendations, resulting in increased adoption and customer satisfaction.
Surfacing New Value-Generating Interventions
XAI not only provides predictions or recommendations but also offers insights into the reasons behind these outcomes. This deeper understanding can help organisations identify hidden business interventions that would otherwise remain unseen. Businesses can intervene effectively and optimise their operations by understanding the underlying factors contributing to predictions.
Ensuring Business Value
By demystifying the black box of AI, XAI enables organisations to ensure that their AI applications are aligned with the intended business objectives. Technical teams can explain how the AI system functions, allowing business teams to confirm that the application delivers the expected value.
Mitigating Regulatory and Other Risks
Explainability helps organisations mitigate risks associated with AI systems. By explaining AI decisions, organisations can ensure compliance with laws and regulations, confirm alignment with internal company policies, and address potential ethical concerns. Clear explanations also help organisations navigate scrutiny from regulators, the media, and the public, reducing the likelihood of reputational damage.
The Challenges of Implementing Explainable AI
While the benefits of XAI are evident, implementing it poses several challenges. The inherent complexity of AI models, especially deep learning and neural networks, makes it difficult to trace the decision-making process. As AI systems continuously learn and update themselves based on new data, the audit trail of insights becomes harder to follow. Additionally, different stakeholders have varied explainability needs, requiring tailored approaches to address their specific concerns.
Overcoming these challenges requires a comprehensive approach to XAI implementation.
Strategies for Implementing Explainable AI in the Banking Industry
To successfully implement XAI in the banking industry, organisations must establish a framework for trustworthy AI, understand explainable AI techniques, and adhere to clear regulations and guidelines.
Establishing Trustworthy AI
Trustworthy AI encompasses privacy, robustness, and explainability. Financial institutions must develop a governance framework that addresses these components and instils trust at every level of the AI process. This includes new ways of collecting and analysing data, ensuring privacy protections, robustness against biases, and implementing explainable AI techniques to facilitate transparency and accountability.
Understanding Explainable AI Techniques
Implementing explainable AI techniques is essential for demystifying the black box of AI decision-making. Stakeholders involved in AI systems should understand how these techniques work and how to interpret the explanations provided by the AI models. Assessing the level of stakeholder comprehension required and making AI platforms explainable to relevant parties, such as engineers, legal teams, compliance officers, and auditors, is crucial.
Creating Clear Regulations and Guidelines
Collaboration between governments, industry, and academia is necessary to regulate the risks associated with AI systems. Governments actively release guidelines and regulations to ensure AI systems' fairness, transparency, and safety. Organisations should actively engage in these regulatory initiatives, adopting standards and tools to verify the reliability of AI models and mitigate unintentional biases.
Conclusion
Explainable AI (XAI) is vital for shedding light on the 'black box' of AI decision-making in the banking industry. By implementing XAI techniques, financial institutions can enhance transparency, build trust, surface new value-generating interventions, ensure business value, and mitigate regulatory and other risks. Overcoming the challenges associated with implementing XAI requires a comprehensive approach encompassing trustworthy AI, understanding explainable AI techniques, and adherence to clear regulations and guidelines.
By embracing XAI, banks can unleash the full potential of AI while fostering trust and accountability in their decision-making processes.
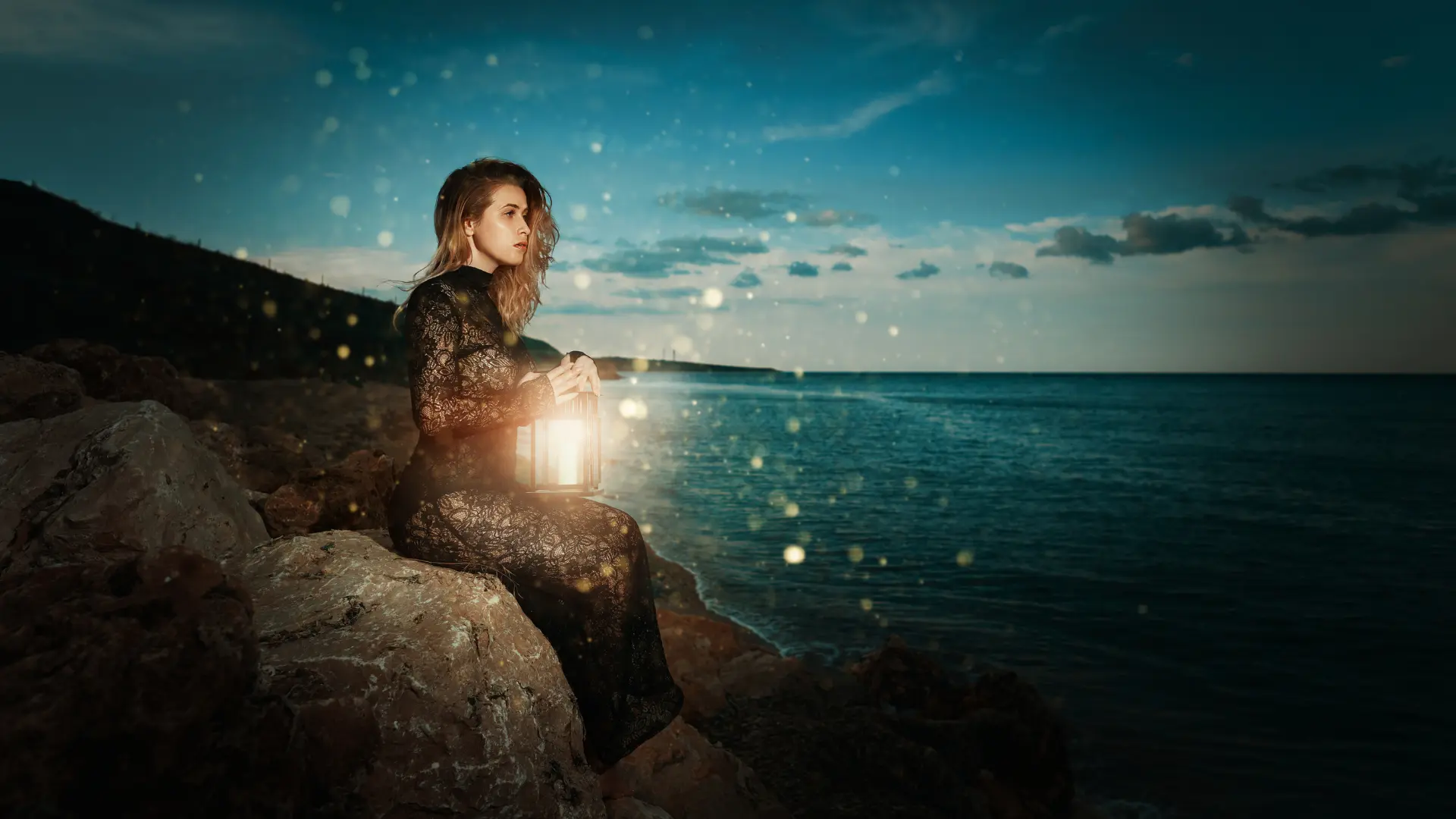